#CycleGAN
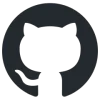
CycleGAN
Discover CycleGAN's approach to unpaired image-to-image translation through cycle-consistent GANs. This technique transforms images independently of matching input-output pairs, enabling new artistic style possibilities such as converting Monet paintings to modern photography or altering seasons and objects. Compatible implementations in PyTorch and Tensorflow, with several pre-trained models, are included for immediate use and testing. Find comprehensive setup and training guides to apply this innovative image translation method in both creative and practical contexts.
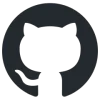
contrastive-unpaired-translation
Discover efficient methods in unpaired image-to-image translation with patchwise contrastive learning. The approach avoids complex loss functions and inverse networks, leading to faster, resource-efficient training compared to CycleGAN. It can be applied to single image training with high-quality results, suitable for a variety of applications. Key benefits include memory efficiency and improved distribution matching, developed collaboratively by UC Berkeley and Adobe Research, and presented at ECCV 2020.
Feedback Email: [email protected]