#depth estimation
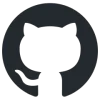
monodepth2
Explore Monodepth2's PyTorch-based reference implementation for advanced depth estimation. The project methodically outlines dependency setups, guides on predicting scaled disparities, and furnishes ready-to-use pretrained models. Detailed instructions for custom dataset training, model finetuning, and KITTI dataset evaluation are included, alongside support for multiple training modalities including monocular, stereo, and combined approaches, catering to diverse research requirements.
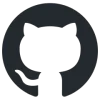
UniDepth
UniDepth provides universal metric depth estimation from single images, accommodating adaptable input shapes, confident predictions, and swift processing. This leading model in depth estimation boasts a strong decoder design and ONNX compatibility, excelling in tests like KITTI and NYUv2. The project progresses with compact model versions and continual image processing enhancements. It facilitates seamless model access via Hugging Face and TorchHub, fostering integration in various AI frameworks. Researchers are encouraged to contribute to this innovative tool, advancing automated visual comprehension.
Feedback Email: [email protected]