#Fine-Tuning
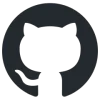
LLMtuner
LLMTuner provides an efficient solution for adjusting large language models, such as Whisper and Llama, using sophisticated techniques like LoRA and QLoRA. Featuring a user-friendly, scikit-learn-inspired interface, it facilitates streamlined parameter tuning and model deployment. The tool offers effortless demo creation and model deployment with minimal code, making it suitable for researchers and developers seeking fast and reliable ML outcomes. Its future features, including deployment readiness on platforms like AWS and GCP, are designed to significantly enhance model training and deployment capabilities.
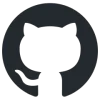
LLMsNineStoryDemonTower
This article offers a thorough analysis of the practical uses of large language models across fields including natural language processing, efficient fine-tuning techniques, and artifact production. It highlights models such as ChatGLM2 and ChatGLM3, and techniques like distributed training and text-to-image conversion. The guide also delves into progress in visual question answering and automatic speech recognition, providing insights into how LLMs are innovating sectors like finance, healthcare, and education.
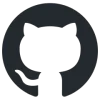
ms-swift
SWIFT provides scalable infrastructure for training, inference, evaluation, and deployment of over 350 Large Language Models and 100 Multimodal Models. It ensures seamless workflow management from development to application with its advanced Adapters library, integrating cutting-edge techniques like NEFTune and LoRA+. Featuring a user-friendly Gradio interface, SWIFT is suitable for both research and production environments. It includes comprehensive documentation and an active community for support, available through Discord and ModelScope Studio.
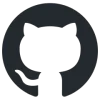
LLM-Adapters
Discover a framework designed for efficient fine-tuning of advanced large language models via a variety of adapter techniques. This framework, supporting models such as LLaMa, OPT, BLOOM, and GPT-J, enables parameter-efficient learning across multiple tasks. It offers compatibility with adapter methods like LoRA, AdapterH, and Parallel adapters, enhancing the performance of NLP applications. Keep informed about the latest achievements, including surpassing benchmarks such as ChatGPT in commonsense and mathematics evaluations.
Feedback Email: [email protected]