#pose estimation
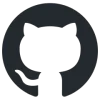
boxmot
BoxMOT offers state-of-the-art, flexible multi-object trackers for segmentation, detection, and pose estimation, adaptable to various hardware from CPUs to GPUs. Compatible with YOLO models, it integrates advanced ReID systems and reduces experimentation overhead with efficient data handling.
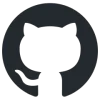
awesome-6d-object
This list provides extensive resources concerning 6D object pose estimation and single-view 3D object reconstruction, plus 3D hand-object pose estimation. It excludes geometry-based methods but spotlights influential papers, open-source demos, and key research. Included are major conference works, 3D vision and robotics workshops, datasets, and relevant challenges for both researchers and developers. Additionally, it features esteemed arXiv papers and resources from premier conferences such as CVPR and ICCV.
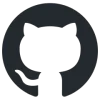
ultralytics
Explore up-to-date tools for object detection and image processing with YOLO11, a cutting-edge model known for its rapid speed, accuracy, and versatility. This model supports a variety of tasks such as object detection, tracking, instance segmentation, image classification, and pose estimation. Building on the success of prior YOLO models, it delivers enhanced performance and intuitive features. Access detailed documentation, community forums, and installation options for seamless project integration. Engage with the developer community for further insights and practical applications of YOLO11.
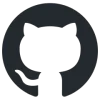
RSN
Discover how Residual Steps Network (RSN) advances multi-person pose estimation by effectively aggregating intra-level spatial features for precise keypoint localization. The Pose Refine Machine (PRM) enhances this precision by refining keypoint positions. As a winner of the COCO 2019 Keypoint Challenge, RSN demonstrates leading performance on both COCO and MPII benchmarks. Now part of the MMPose framework, RSN offers a substantial resource for pose estimation research and development.
Feedback Email: [email protected]