#Self-supervised Learning
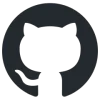
awesome-self-supervised-gnn
Discover a curated compilation of research papers on self-supervised learning in graph neural networks (GNNs), organized by year. This resource includes widely-cited studies and offers access to related code for further exploration. Stay informed on recent developments, suggest additions, or report errors. Topics covered include community detection, representation learning, and anomaly detection, with insights into pioneering techniques and applications.
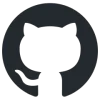
UNI
This article introduces a major advancement in computational pathology with the UNI model, a self-supervised model trained on a vast dataset of over 100 million histopathology images across 20 different tissue types. It addresses the complexities of annotating high-resolution whole-slide images and excels in 34 clinical tasks, including resolution-agnostic tissue classification and few-shot classification of up to 108 cancer types. Learn how UNI enhances data efficiency and adapts to clinical workflows, surpassing existing models in performance across diverse diagnostic challenges.
Feedback Email: [email protected]