#training data
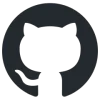
snorkel
Discover a groundbreaking method in machine learning that emphasizes efficient data labeling and management to reduce manual labor. Originating from Stanford, this project has joined forces with tech giants like Google and Intel, contributing to over sixty peer-reviewed publications and supporting real-world applications. With Snorkel Flow, the project advances into a comprehensive AI platform, incorporating cutting-edge techniques in weak supervision, data augmentation, and multitask learning, offering researchers and practitioners a streamlined process for AI development.
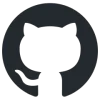
LLaVA-Plus-Codebase
LLaVA-Plus enhances the functionalities of language and vision assistants by incorporating tool use for vision tasks. It forms multimodal agents capable of learning and utilizing various skills. The project's design simplifies installation and ensures operating system compatibility, offering specific guidance for Linux, macOS, and Windows. Detailed demo setups and training guides enable model deployment and utilization of public checkpoints in the Model Zoo. The training is structured in two stages: feature alignment and tool-enhanced visual instruction tuning, utilizing massive datasets like COCO and VisualGenome. Available for research purposes under defined licenses, the project supports the advancement of multimodal AI by permitting non-commercial use.
Feedback Email: [email protected]