#3D reconstruction
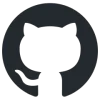
ICON
Discover how ICON advances 3D human reconstruction using implicit surfaces, integrating with PyTorch and Hugging Face. It extracts detailed 3D clothed models from single RGB images, offering robust pipelines like PIFu, PaMIR for effective training and evaluation. Understand its advanced garment extraction, pose refinement, and normal prediction technologies, supporting frameworks like SMPL-X for enhanced 3D modeling with detailed garment features and dynamic video outputs.
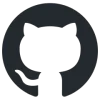
Wonder3D
Wonder3D converts single-view images into detailed 3D meshes in minutes using cross-domain diffusion. It achieves high-quality reconstruction by creating consistent multi-view normal maps and employing a novel fusion method. The project offers regular updates, code resources, and examples to support users and invites collaborations for further enhancement.
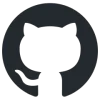
Instant-angelo
Instant-angelo facilitates the creation of high-fidelity digital replicas from RGB videos in under 20 minutes. It integrates UniSDF for improved reflective surface reconstruction, addressing challenges in the rapid 3D modeling of both small and large scenes. The installation is straightforward, supporting modes like smooth and detailed reconstruction to cater to various needs. Although tools such as COLMAP are initially required, they enhance result optimization. Ideal for diverse applications like virtual tours, this project offers an efficient solution for precise neural reconstructions.
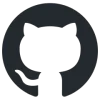
toon3d
Toon3D facilitates 3D scene reconstruction from 2D cartoons, overcoming geometric inconsistencies. Through a precise image processing pipeline, it achieves visually coherent structures, paving new pathways in animation and virtual reality. Steps include setting environments, dataset management, and depth-based data processing, leading to potential advancements in computational art.
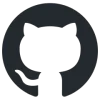
vggsfm
Discover cutting-edge 3D reconstruction strategies using VGGSfM, which combines deep learning for structure-from-motion with dynamic sequence analysis. The project facilitates dense point cloud generation and camera pose recovery from sequential data frames. Recent enhancements include functionalities like dense depth map export and Gaussian splatting model training. Optimized for processing over 1,000 video frames and adept at filtering dynamic objects via masks, VGGSfM serves as a precise tool for practitioners and developers in the fields of computer vision and augmented reality.
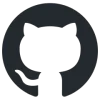
Cycle3D
Examine how Cycle3D applies a generation-reconstruction cycle to achieve quality image-to-3D conversion. Utilizing a 2D diffusion model and a reconstruction model, it ensures consistent multi-view generation. Learn more about Cycle3D's method to manage intricate processes efficiently and access updates via their project page and arXiv paper. Keywords: image-to-3D generation, texture quality, consistency.
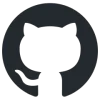
TripoSR
TripoSR is an open-source tool designed for efficient 3D reconstruction from a single image, developed by Tripo AI and Stability AI. It implements the Large Reconstruction Model approach to achieve fast processing and superior quality, producing models in under 0.5 seconds using NVIDIA A100 GPUs. TripoSR has shown better performance than other open-source models on several datasets. The project, distributed under the MIT license, provides source code, pre-trained models, and a user-friendly demo, facilitating advancements in 3D generative AI for researchers and developers.
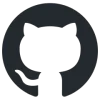
awesome-6d-object
This list provides extensive resources concerning 6D object pose estimation and single-view 3D object reconstruction, plus 3D hand-object pose estimation. It excludes geometry-based methods but spotlights influential papers, open-source demos, and key research. Included are major conference works, 3D vision and robotics workshops, datasets, and relevant challenges for both researchers and developers. Additionally, it features esteemed arXiv papers and resources from premier conferences such as CVPR and ICCV.
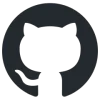
Real3D
Real3D utilizes single-view real-world images to efficiently train large reconstruction models. Its unique self-training framework with unsupervised learning enables detailed supervision without ground-truth 3D data. The project introduces an automatic data curation method, enhancing data quality and diversity for better performance across diverse scenarios. Real3D effectively combines synthetic and real-world data for more accurate 3D modeling.
Feedback Email: [email protected]