#Dataset
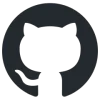
LISA
LISA employs a large language model to enhance segmentation tasks, particularly in reasoning segmentation through complex and implicit queries. It uses a detailed benchmark of image-instruction pairs, encompassing extensive world knowledge to provide detailed answers and supports multi-turn dialogue. Demonstrating strong zero-shot learning ability, LISA performs well on datasets without reasoning data, and fine-tuning even with limited data boosts its performance. LISA achieved notable recognition at CVPR 2024. Discover LISA's efficiency through our online demo.
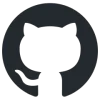
PerceptualSimilarity
Discover how LPIPS leverages deep learning to assess image similarity with a focus on perceptual differences. Employing the PyTorch framework, it provides a novel approach to image patch comparison, surpassing traditional techniques. The project also includes the BAPPS dataset for comprehensive evaluation and training, supporting network architectures such as SqueezeNet, AlexNet, and VGG. This tool is valuable for researchers interested in cutting-edge image analysis methodologies.
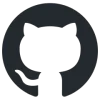
Calliar
Discover Calliar, a rare online dataset specifically for Arabic calligraphy, with 2,500 annotated files that provide detailed insights into strokes, characters, words, and sentence structures. This dataset effectively fills a gap in Arabic calligraphy resources by offering advanced visualization tools and data for both research and artistic endeavors. Stored in json and npz formats, it ensures efficient storage and accessibility for scholars and enthusiasts working within digital spaces. Complete with visualization scripts and server setup instructions, Calliar delivers a full toolkit for researchers and calligraphy aficionados alike.
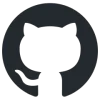
audio2photoreal
This project provides tools for generating photorealistic human avatars in conversations using audio inputs. It includes PyTorch-based resources, with training/testing codes and pretrained models. A demo is available for trial, and code can be run locally for further exploration. This tool is suited for those interested in human-computer interaction, speech processing, and virtual reality, focusing on synthesizing body language and facial expressions.
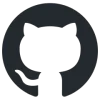
speech_dataset
Explore a diverse collection of speech datasets in multiple languages, including Chinese, English, and Japanese, designed for speech recognition, synthesis, and speaker diarization. This collection supports various applications, such as speech commands and ASR system evaluation, facilitating advancements in speech technology. Notable datasets like Common Voice and LibriSpeech play a crucial role in enhancing machine learning models. This resource is invaluable for researchers seeking comprehensive audio data for developing speech-related solutions across different linguistic contexts.
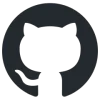
xtts2-ui
The XTTS-2-UI project provides a straightforward interface for cloning voices in 16 languages using text and a brief audio sample. The model tts_models/multilingual/multi-dataset/xtts_v2 is automatically downloaded when first used, aiding in seamless voice cloning experiments. It supports both voice recording and uploading with a few setup steps. The application can operate via terminal or Streamlit, requiring agreement to the terms of service initially.
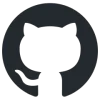
GigaSpeech
GigaSpeech is a significant ASR corpus consisting of 10,000 hours of transcribed audio designed for a broad range of speech recognition applications. The dataset continually evolves to support numerous speech recognition toolkits like Kaldi and ESPnet, ensuring easy data preparation. Featuring contributions from major institutions, it offers rich audio sources including audiobooks, podcasts, and YouTube content suitable for both supervised and semi-supervised learning. With detailed metadata and resampling guidelines, it aims to extend ASR features, supporting future tasks such as speaker identification and language diversification. A valuable resource for researchers and developers in need of a comprehensive audio dataset.
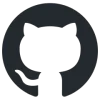
Few-NERD
Discover Few-NERD, a detailed dataset for named entity recognition featuring 8 broad categories and 66 detailed entity types. This valuable resource supports supervised and few-shot learning with three benchmark tasks, encompassing 188,200 sentences and around 500,000 entities. Easy BERT integration facilitates advanced training, and regular updates ensure relevance for researchers addressing complex natural language processing problems.
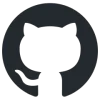
ml-ferret
Ferret is a comprehensive Multimodal Large Language Model (MLLM) designed for various referring and grounding tasks. Innovations include Hybrid Region Representation and Spatial-aware Visual Sampler. It comes with the GRIT Dataset for robust instruction tuning and the Ferret-Bench evaluation benchmark. Components like Ferret-UI and model checkpoints illustrate its proficiency in handling complex tasks, serving the research community within the scope of licensing agreements.
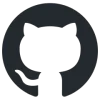
Amphion
Amphion is an open-source toolkit designed to enhance reproducible research in audio, music, and speech generation. It offers essential features such as text-to-speech and singing voice conversion, making it a useful tool for researchers. The toolkit includes high-quality vocoders and evaluation metrics, facilitating advanced audio signal production. Amphion's platform enables the transformation of diverse inputs into audio and aids in developing large-scale speech synthesis datasets.
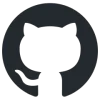
ScreenAgent
ScreenAgent enables Visual Language Model agents to interact with computer interfaces effectively through structured task breakdowns and executions. Utilizing the VNC protocol ensures broad OS compatibility. The comprehensive ScreenAgent dataset supports diverse task automation, highlighting a methodical approach rather than a revolutionary change.
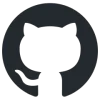
LLIE_Survey
This paper conducts an extensive survey on low-light image and video enhancement, describing advanced techniques and presenting datasets like SICE_Grad, SICE_Mix for complex scenarios, and Night Wenzhou for diverse aerial views. It addresses recent updates, including key revisions, improved images, and metric scripts to support research and application. Covering progress from traditional to deep learning methods, it provides downloadable datasets and benchmark references. The list of models and experiments showcases the developments in this field, serving as a crucial resource for understanding or improving low-light conditions in digital imagery.
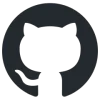
SAM-Med2D
Delve into SAM-Med2D, an expansive and varied dataset crafted for 2D medical image segmentation, including 4.6 million images and 19.7 million masks. Designed to refine models, it covers 10 data modalities and a multitude of anatomical structures. Through sophisticated enhancements to the Segment Anything Model (SAM), this initiative pioneers advancements in medical imaging segmentation, providing notable gains in accuracy and operational efficiency. Keep abreast of continuous updates and potential collaborations in propelling the field of medical AI forward.
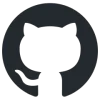
Step-DPO
Step-DPO improves reasoning in language models via a step-wise preference framework, using a robust 10K-step dataset. It enhances models like Qwen2-7B-Instruct, raising MATH performance by 5.6% and GSM8K by 2.4% with limited data. The method yields 70.8% and 94.0% on Qwen2-72B-Instruct for MATH and GSM8K tests, outperforming models like GPT-4-1106. Suitable for researchers and developers, Step-DPO includes a demo and detailed documentation for easier implementation and evaluation.
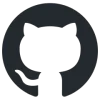
rulm
Discover significant developments in Russian language models through efficient implementations and detailed comparisons. Featuring the RuTurboAlpaca dataset with GPT-3.5-turbo and the Saiga models, the project provides valuable resources on HuggingFace and GitHub. It enables interaction with models from 7b to 70b, fostering innovation in Russian NLP tasks with active community support via DataFest and fine-tuning in Colab.
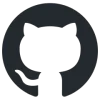
LangSplat
LangSplat employs Gaussian splatting for 3D language model generation, integrating language features into SfM datasets. The project includes a PyTorch-based optimizer and scene-wise autoencoding, offers pre-trained models, and datasets like 3D-OVS and LERF, facilitating 3D scene processing innovations. It supports 3D object localization and semantic segmentation.
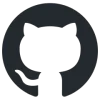
OCR_DataSet
This resource provides a wide collection of OCR datasets specifically for detection and recognition purposes, standardized for easier use. The datasets include well-known names such as ICDAR2015, MLT2019, and COCO-Text_v2, and are available for download from Baidu Cloud. These datasets support multiple languages and offer comprehensive annotation formats ideal for training and evaluating OCR models. Additionally, it includes scripts for data reading, making it a valuable tool for researchers and developers in the field of optical character recognition.
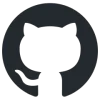
plinder
PLINDER provides a detailed dataset of over 400,000 protein-ligand interaction systems, each with over 500 annotations, crucial for docking algorithm development and assessment. It synchronizes with the Protein Data Bank, includes 14 metrics along with broad similarity scores, and is crafted by eminent organizations including the University of Basel and NVIDIA. It serves as a benchmark for interaction datasets, offering structured splits and reliable evaluation for model comparison. Users can engage with the growing community and apply PLINDER for setting benchmarks and fostering innovation in machine learning and structural biology contests.
Feedback Email: [email protected]