#machine translation
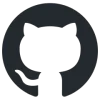
alpaca-chinese-dataset
Discover the Alpaca-Chinese Dataset, using machine translation and self-instruction to fine-tune instruction data. Following the JSON format of original Alpaca data, it offers structured Chinese instructions, useful for tasks like identifying airports or listing fruits, aiding NLP and AI model development.
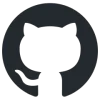
auto-novel
Experience machine translations of Japanese novels effortlessly. This platform allows browsing popular sites like Kakuyomu and Pixiv, or uploading EPUB/TXT files for translation with tools such as Baidu, GPT-3.5, or GPT-4 API. It supports various formats, including bilingual views, and facilitates the creation of EPUB and TXT files for ease of reading. Participate in the community by contributing to project development. Access Japanese literature seamlessly with advanced translation technology.
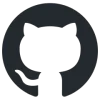
tensor2tensor
Tensor2Tensor is a library developed by Google Brain for deep learning, providing a range of models and datasets to enhance machine learning research. Now succeeded by Trax, T2T supports various tasks like image classification and language translation. Its architecture facilitates easy integration and modification of models, datasets, and hyperparameter sets. Highlighting models such as the Transformer and datasets like ImageNet and MNIST, it supports multiple modalities including text, audio, and images. Tensor2Tensor serves as a versatile tool for developers to innovate and build advanced AI solutions.
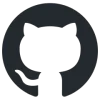
translation-agent
This Python demonstration presents a unique approach to machine translation through a reflection-based agentic workflow. Utilizing a large language model (LLM) for translating and refining texts, the system offers customization in style and terminology usage. While not always surpassing commercial tools, its potential for superior translation warrants further exploration. Open-source contributions and discussions are encouraged to advance this promising translation technology.
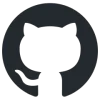
COMET
Discover COMET's capabilities in improving translation precision via context use and error identification. Recent updates introduce XCOMET models for refined error classification by MQM typology, and DocCOMET for document-level context evaluations. This system supports discourse phenomena and chat translation quality assessments. Learn about the models and their roles in enhancing machine translation evaluations.
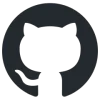
seamless_communication
Seamless is an AI-driven multilingual and multimodal translation project that supports extensive language coverage and promotes authentic interactions. The SeamlessM4T model underpins the broader ecosystem, including SeamlessExpressive and SeamlessStreaming, allowing for real-time, expressive translations and simultaneous translation capabilities in around 100 languages. Seamless utilizes the innovative UnitY2 architecture to improve translation efficiency and reduce latency.
Feedback Email: [email protected]