#NeurIPS 2024
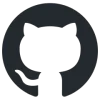
RegionSpot
Discover the 'Recognize Any Regions' project focused on accurate region detection through RegionSpot techniques. The initiative offers straightforward access to source code and pre-trained checkpoints for immediate testing. The RegionSpot model enables mask identification and AP metric computation in both rare and common contexts, with demonstrated efficiency in Box APs and Mask APs. Designed for research and development, the project features demo scripts for easy integration. Checkpoints and code are accessible via Google Drive and OneDrive.
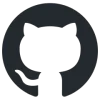
CV-VAE
CV-VAE facilitates the integration of latent generative video models with pretrained models such as SD 2.1 and SVD. Providing comprehensive training and inference resources, it simplifies video reconstruction processes. Compatible with Python 3.8+ and PyTorch 1.13.0+, CV-VAE leverages NVIDIA GPU and CUDA for enhanced performance. Recent developments include its acceptance at NeurIPS 2024 and the availability of new resources for CV-VAE-SD3, further optimizing video processing by aligning with existing architectures.
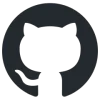
1d-tokenizer
The 1d-tokenizer encodes a 256x256 image into 32 tokens, significantly speeding up the process and achieving approximately 410 times faster results than traditional models while maintaining quality. Accepted by NeurIPS 2024, this project introduces a compact framework that overcomes 2D constraints, enhancing image representation efficiency. It includes updates and multiple model sizes for both VQ and VAE, aiding research advancement in image tokenization with training and evaluation resources.
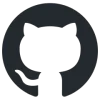
Vista
Vista presents a generalizable model for simulating various driving scenarios with high accuracy. It predicts over long durations, performs complex actions like steering and speed decisions, and offers feedback without ground truth data. Recent updates have improved user accessibility through new model weights, installation guides, and documentation. The online demo showcases its capabilities in simulated environments, advancing autonomous driving technology.
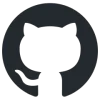
PuLID
Explore sophisticated approaches to ID customization utilizing the contrastive alignment methodologies of PuLID. Investigate efficient GPU-compatible solutions backed by diverse demos and resources. Keep abreast of the latest PuLID models and enhancements like PuLID-FLUX, designed for optimal local and online execution. Enjoy straightforward integration aided by easy installation and detailed guides. Utilize ongoing updates and support for consistent performance advancements. PuLID serves as a significant resource for researchers and developers focused on improving AI-based image generation.
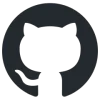
cond-image-leakage
This project investigates the issue of conditional image leakage in image-to-video diffusion models (I2V-DMs), which causes videos to lack dynamic motion. By proposing practical plug-and-play strategies for both inference and training, the project demonstrates improvements using various I2V-DMs like DynamiCrafter, SVD, and VideoCrafter1. Comprehensive setup instructions and parameter optimization insights make this a key resource for improving video generation.
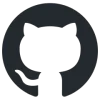
FIFO-Diffusion_public
Delve into innovative methods that transform text into infinite video outputs without training requirements, utilizing minimal VRAM for greater accessibility. The project supports VideoCrafter2 for single GPU use and Open-Sora Plan for distributed inference, catering to varied creative applications with reduced computational load. Uncover advancements in video generation demonstrated at NeurIPS 2024.
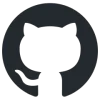
KnowledgeEditingPapers
Delve into key research papers on knowledge editing for large language models, focusing on techniques to efficiently modify model behavior while maintaining overall performance. This collection provides insights into approaches such as memory-based techniques and parameter adaptations, featuring notable advancements by leading researchers. Gain an understanding of critical topics like model updates, bug fixes, lifelong learning, and security and privacy issues within the evolving field of language models.
Feedback Email: [email protected]