#Survey
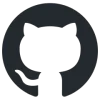
RAG-Survey
Retrieval-Augmented Generation (RAG) is enhancing AI-generated content by integrating retrieval methods with generative AI. This comprehensive survey categorizes research on RAG's foundations and methods, including query-based, latent representation-based, logit-based, and speculative approaches. It highlights advancements in input, retrieval, and generation enhancements and covers applications like open-domain question answering and video captioning. Keep updated with this extensive review on how RAG influences AI content development.
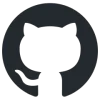
Transformer-in-Vision
This project examines the growing significance of Transformer technology in a variety of computer vision applications. It serves as a comprehensive resource, aggregating recent studies from fields like robotics and image processing, and highlighting the essential role of Transformers in AI models. The project outlines innovations such as LLM-in-Vision, and delivers thorough surveys on complex topics, such as multi-modal pre-training and generative adversarial networks, providing readers with insights into this evolving field.
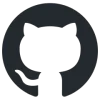
Awesome-LLMs-Evaluation-Papers
This objective overview explores various evaluation methods for large language models, focusing on assessing their knowledge, alignment, and safety. It presents an extensive collection of papers and methods curated by Tianjin University's team and underscores the necessity for meticulous evaluations to mitigate potential risks such as data leaks. The survey aims to foster responsible AI development and maximize societal benefits through a structured approach.
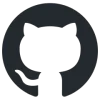
AI-Software-Startups
SSAIS (Survey of Startups on Artificial Intelligence Software) offers a regularly updated collection of AI software startups, detailing companies from infrastructure to applications across countries including China, the USA, and Israel. The repository categorizes startups by technology, offering insights into the latest funding rounds and commercial impact. It aims to highlight the current state and innovation potential in fields such as data processing, NLP, and vision.
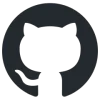
Awesome-LLM-Survey
Discover an extensive compilation of surveys on Large Language Models, addressing critical areas such as instruction tuning, human alignment, and multi-modal integrations. Understand challenges like hallucination and compression, with insights into their applications in domains like health, finance, and others. A valuable tool for researchers involved in LLM development.
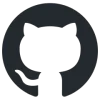
survey
Learn about creating interactive prompts in terminal environments with the Survey library, which offers functionality using ANSI escape sequences. Even though no longer maintained, it includes examples of prompt types like Input, Password, Confirm, and more, and provides options for filtering, validation, and customization. Explore its codebase for educational insights on user feedback management in interactive sessions. For a maintained alternative, see the charmbracelet Bubble Tea library.
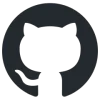
RAG-Survey
This survey explores Retrieval-Augmented Generation (RAG), covering diverse techniques like query and data augmentation, with ongoing updates reflecting the field's growth. It offers insights into advanced applications in content generation and knowledge base enhancement.
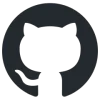
Awesome-Diffusion-Models-in-Medical-Imaging
Explore a curated collection of scholarly articles on diffusion models in medical imaging, featuring survey papers, challenges, and applications including anomaly detection and image restoration. This project compiles influential publications from conferences and journals like Medical Image Analysis and MICCAI 2023, serving as a valuable resource for professionals seeking the latest advancements in diffusion model applications.
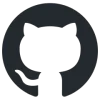
LLIE_Survey
This paper conducts an extensive survey on low-light image and video enhancement, describing advanced techniques and presenting datasets like SICE_Grad, SICE_Mix for complex scenarios, and Night Wenzhou for diverse aerial views. It addresses recent updates, including key revisions, improved images, and metric scripts to support research and application. Covering progress from traditional to deep learning methods, it provides downloadable datasets and benchmark references. The list of models and experiments showcases the developments in this field, serving as a crucial resource for understanding or improving low-light conditions in digital imagery.
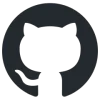
3D-Gaussian-Splatting-Papers
This platform presents an organized collection of 3D Gaussian Splatting research, including papers and reviews from leading conferences like CVPR2024 and ECCV2024. It highlights research across various institutions, offering insights into applications such as robotics and 3D reconstruction. The repository includes resources like code and abstracts, supporting further exploration. This centralized database helps users stay updated on advancing technologies in 3D Gaussian Splatting.
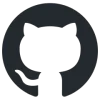
KG-LLM-Papers
This repository provides a comprehensive collection of academic papers on the integration of Knowledge Graphs (KGs) and Large Language Models (LLMs). It is a valuable resource for researchers and practitioners interested in advancing domain-specific applications, improving question answering, and enhancing reasoning through seamless KG-LLM integration. Contributions are welcomed via Issues or Pull Requests to expand this growing database. Stay informed about the latest advancements, preprints, and surveys on KG and LLM interactions. Explore resources aimed at minimizing hallucinations, improving retrieval, and enhancing factuality in AI solutions.
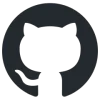
ABigSurvey
This survey compiles and organizes over 1063 papers on NLP and ML, offering an analysis of trends by following ACL and ICML submission guidelines. It covers topics like computational social science, dialogue systems, information extraction, and more. Graphs and word clouds display research distribution, focusing on significant areas such as large language models and generative adversarial networks. It is a useful resource for understanding the landscape and future directions in NLP and ML.
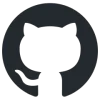
ABigSurveyOfLLMs
This survey offers an expansive view of recent developments in large language models (LLMs) within artificial intelligence. It aggregates a significant number of research papers from diverse conferences and open-access resources, providing an extensive review of the LLM field. Topics covered include alignment, data management, societal implications, and applications in sectors like healthcare and education. Key challenges such as safety, misinformation, and efficiency are also examined. This compilation is intended to assist researchers and practitioners in gaining a rapid understanding of the field and in exploring emerging research pathways in LLMs.
Feedback Email: [email protected]